Enhancing Social Sciences Research with Data Analysis through Arabic Tweets
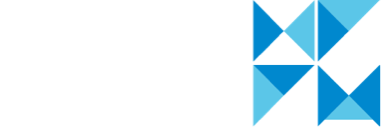
- Client Hamad Bin Khalifa University
- Industry Education
-
Solution
- Automated annotation
- Document PDF
The automated annotation solution provided by aiXplain has significantly enhanced our research capabilities at Hamad Bin Khalifa University. The accuracy and efficiency of the annotated Arabic tweets have provided us with reliable data, allowing us to advance our social sciences research with confidence. This project has not only improved the quality of our research but has also saved us valuable time and resources. We are extremely pleased with the results and look forward to future collaborations.
Dr. Wajdi Zaghouani, Associate Professor in Digital Humanities
Hamad Bin Khalifa University commissioned a project utilizing labeled tweets to support data analysis in social sciences research. The primary goal was to annotate approximately 20,000 Arabic tweets to detect emotions and offensive content. However, the project faced significant challenges, particularly in ensuring the quality of annotations due to the diverse nature of Arabic dialects.
Challenge
The project’s main challenge is conducting quality checks on the annotated tweets. With a large volume of tasks to handle, it was impractical to review each one individually. Moreover, the inherent diversity in Arabic dialects posed a substantial obstacle, making it difficult for annotators to determine whether tweets were offensive across various cultural backgrounds accurately.
Solution
To address these challenges, a solution involving implementing an inter-annotator agreement (IAA) process was devised. Annotators were tasked with annotating an additional set of 250 tweets, which were then subjected to IAA analysis. This process aimed to assess the consistency of annotations among different annotators on the same data. Based on the IAA results, top-performing annotators were identified, while those with lower scores were excluded from further tasks. Subsequently, incorrectly annotated tasks were redistributed to annotators with higher IAA scores for completion.
The IAA significantly boosted the accuracy of the labeled dataset, ensuring more reliable results for social sciences research. By identifying top-performing annotators and redistributing tasks accordingly.
Impact
Implementing the inter-annotator agreement improved the accuracy with which Arabic tweets were labeled, making the data more reliable for social sciences research. It also helped save time and resources by better managing who did what tasks.
Conclusion
In conclusion, adding more annotation tasks and using the inter-annotator agreement helped determine which Arabic tweets were offensive. This improved the data and also saved time and resources.