Ethics in AI: Navigating the Moral Landscape of Artificial Intelligence
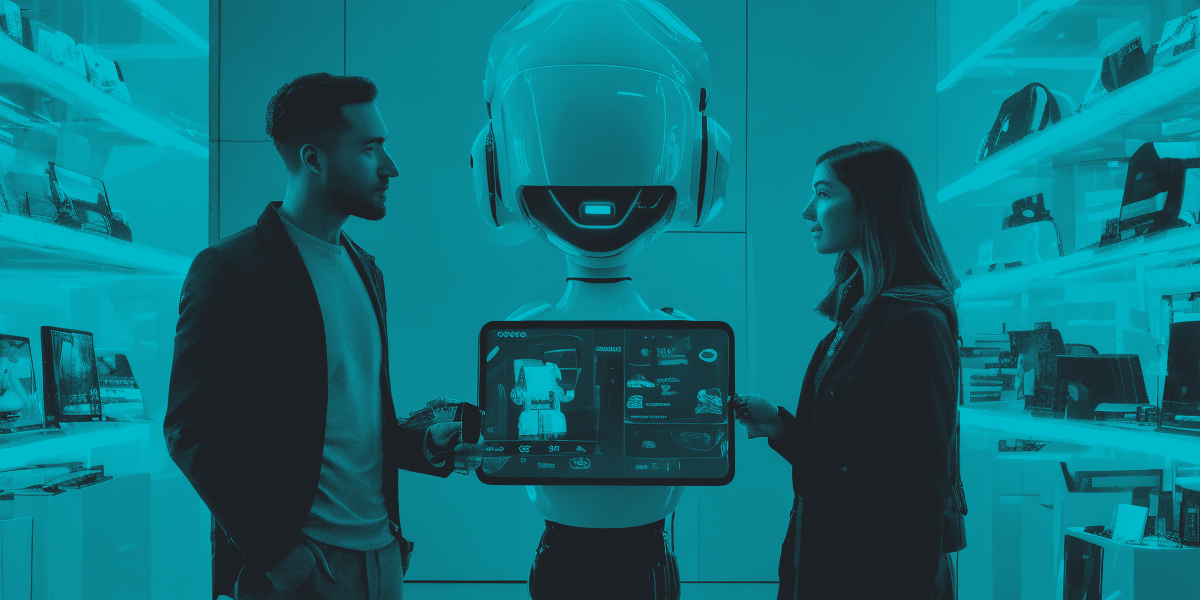
“With great power comes great responsibility.” This famous quote from Ben Parker is a succinct and wholesome representation of what ethics needs to be for artificial intelligence. As Large Language Models (LLMs) continue to advance at an unprecedented pace and reshape how we live and work, it becomes imperative to allocate the same amount of resources to developing a standardized code of ethics as we have to R&D.
Bias and Fairness: The Challenge of Algorithmic Prejudice
Bias in AI algorithms is a pressing concern. AI systems are trained on extensive amounts of data and require preprocessing. That in itself is a feat. But the point of concern, the actual cleansing of the content, is often overlooked. For example, the GPT3 – a first-of-its-kind language model that put the power of AI in the hands of the masses – the model wasn’t exactly “pure,” as it was trained using data containing profanity, racial stereotypes, and gender bias. While the latest version, GPT 4, has been represented as less biased, recent studies have shown that outputs still contain partisan language and stereotypes.
Privacy and Data Protection: Safeguarding Personal Information
AI systems often rely on extensive data collection to function effectively. However, the use of personal data without proper consent or for unintended purposes opens a pandora’s box of concerns about individual privacy. High-profile data breaches have highlighted the importance of robust data protection measures.
To uphold ethical standards, organizations must comply with data protection regulations, such as the General Data Protection Regulation (GDPR) in the European Union. Implementing privacy by design principles and obtaining explicit consent from users are essential steps to protect personal information in AI applications.
It All Comes Down to Accountability
Maybe the scientists and engineers had good intentions, but they lacked in the implementation. Without the proper checks in place, our models aimed at helping others can actually have the opposite effect. I liken the situation to the popular show Doctor Who. The Doctor goes around traveling at various points in time and space. He has a wealth of knowledge that he possesses combined with lots of power, but if that goes unchecked, then he can drive himself mad, or worse, hurt others. He needs a companion that can be present to humanize him and ensure that he does not take his power too far. When we have a large model, like an imaging, speech or text model, we need to be able to humanize them.
In the past few years, more has been done to advance the notion of responsible AI and AI ethics than ever before. The recent NeurIPS conference initiated the first of its kind ethics evaluation panel which oversaw the final submission of AI models by evaluating the pros and cons of deploying the submitted models in industry. Moreover, the AI ethics committee from Google (the committee was disbanded, and raised some issues.) proposed a viable approach to enact accountability. They propose that researchers need to submit model cards with their models. Each model should have a document explaining what the model is, what it is used for, what are the known limitations, the trade-offs, and any issues with specific demographic groups. As long as this exists, transparency also exists, and researchers are more accountable in those aspects.
Of course, these are things that can be implemented to subdue the negatives of AI that exist within models and in the hearts of consumers that do not trust AI for its fallacies, but also do not quell them. For a long time, technology has been able to avoid accountability, and this can be attributed to the lack of proper regulation in the technology industry. The famous show Silicon Valley actually mentioned a term called “tethics”, and it is a point of research also being proposed by faculty at UC Berkeley. The idea is that there should exist a binding code of ethics in technology in a similar way in which all doctors must uphold the Hippocratic Oath. If anything can cure the negative stigma around AI, it will be through “tethics” and accountability.
Conclusion
The ethical implications of AI are vast and multifaceted. A collective effort is required to address them responsibility. By embracing transparency, fairness and human-centric design principles, we will be able to navigate the moral landscape to create a code of ethics and public policy that promotes the positive effects AI can have on society.
References
[1] Prestigious AI meeting takes steps to improve ethics of research
[2] Are We Automating Racism?
[3] Lecture 9: AI Ethics
[4] Ethics in AI research papers and articles
[5] A Practical Guide to Building Ethical AI
[6] Gender Shades: Intersectional Accuracy Disparities in Commercial Gender Classification
[7] Addressing Bias in GPT-4: Ensuring Fair and Equitable AI